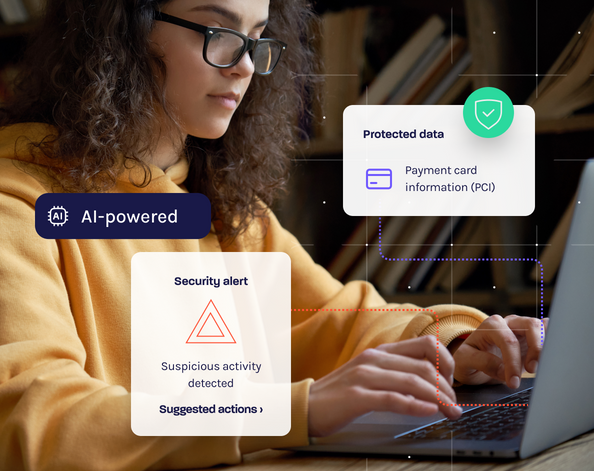
Fraud Detection and Machine Learning: A Proactive Approach
Financial institutions face an escalating battle against credit card fraud, identity theft and payment manipulation—schemes that drain billions annually. As digital services expand, fraudsters exploit more entry points, forcing banks and insurers to ramp up defenses while preserving customer trust. But while fraud grows more sophisticated, you can fight back with equally advanced tools powered by artificial intelligence.
Machine learning (ML) delivers a proactive approach to identify and prevent suspicious activity before it escalates. Unlike static rules or manual reviews, robust machine learning models continuously learn from user behavior, transaction logs and other data streams. These insights detect subtle shifts in activity patterns, letting you intercept threats earlier and with sharper accuracy. It’s why AI-driven fraud detection now anchors modern financial fraud prevention strategies.
In this blog, we’ll explore how fraud detection and machine learning combine to fortify security. We'll also break down key ML techniques, real-world applications, future trends and actionable steps to safeguard your organization.
How Machine Learning Transforms Fraud Detection
Machine learning expands on traditional fraud management by giving financial institutions the adaptability and precision needed to keep pace with changing threats. Rather than applying fixed rule sets that can quickly become outdated, machine learning algorithms learn from new data and spot abnormal behaviors in real time. This adaptability, coupled with pattern recognition, makes AI-driven fraud detection critical for defending digital channels against increasingly cunning fraudsters.
ML models examine vast volumes of information—such as clickstream events, API calls and transaction data—to identify deviations from typical usage. When unusual patterns emerge, these systems automatically flag potential threats for further review. This data-driven approach cuts down on the guesswork and subjectivity that often lead to oversights or false positives in manual reviews.
By leveraging advanced analytics, solutions like Glassbox configure dynamic metrics tailored to your specific environment, using insights drawn from every customer journey to provide unparalleled fraud detection capabilities.
There are three primary approaches to machine learning in fraud prevention:
1. Supervised Machine Learning for Fraud Detection
Supervised learning uses historical cases of confirmed fraud to teach a machine learning model what malicious behavior looks like. Through labeled examples of legitimate transactions and fraudulent activities, the model learns to recognize fraud patterns more accurately over time. It’s especially common in credit card fraud detection, where banks regularly train these models on known fraudulent transactions to block suspicious payments.
Identity verification also benefits from supervised approaches that compare new account signups against documented fraud cases. Many organizations pair supervised models with Glassbox’s deep digital journey analysis, so they can quickly label and classify events for real-time risk scoring.
2. Unsupervised Learning for Emerging Threats
Unsupervised learning doesn’t rely on predefined labels. Instead, it groups similar behaviors or transactions into clusters, highlighting events that deviate from the typical patterns. This is especially useful for new or emerging fraud tactics that haven’t been labeled in past datasets. Unsupervised algorithms can adapt by continually re-clustering behaviors—revealing anomalies like a spate of account takeovers or suspicious field-level manipulations. Glassbox’s analytics platform amplifies these insights with the ability to surface hidden anomalies across every digital touchpoint, giving teams an early warning system for unexplained deviations.
3. Deep Learning for Complex Pattern Recognition
Deep learning models are designed to analyze sequences, such as user session flows and transaction logs, to spot subtle indicators of fraud. Unlike simpler algorithms, these neural networks automatically generate features from unstructured data, which helps them capture sophisticated techniques used by fraudsters. Deep learning is particularly advantageous in high-stakes environments where criminals constantly evolve their methods. Glassbox enriches these models by mapping every interaction in sprawling user journeys, contrasting legitimate flows with fraud’s telltale breadcrumbs.
The real power lies in combining all three approaches. But how does it stack up against traditional fraud detection methods?
Traditional Fraud Detection vs. Machine Learning
Traditional fraud detection leans on manual reviews and rigid rules—which can often trigger false positives. In these systems, fraud analysts must sift through daily volumes of suspicious activity, attempting to verify each item by hand. And because the rules rarely update in real time, many organizations find themselves reacting after the damage has been done. This causes costs to climb as outdated methods struggle to match evolving fraud patterns.
Static, rules-based systems also tend to be brittle. Fraudsters get wise to any known thresholds or filters, adjusting their methods to sneak past detection. Because there’s no built-in learning, these systems remain largely reactive. Moreover, setting conservative thresholds can create an avalanche of alerts, draining valuable analyst hours and frustrating legitimate customers.
Machine learning flips the script. Algorithms adapt continuously, fueled by new data and feedback—not one-size-fits-all rules. They align with your unique risk profile, transaction patterns and fraud trends. Anomalies stand out instantly—even unknown threats—flagging risks earlier while sparing legitimate activity from unnecessary scrutiny.
The result? Fewer false positives, smoother customer journeys and analysts freed from chasing ghosts.
AI-driven solutions shine with continuous learning: models ingest fresh data from web, mobile and backend systems, refining detection as fraudsters pivot. This omnichannel data support is essential in today’s environment, where fraudsters aim to exploit any gap—be it an API endpoint or a payment gateway.
Real-time alerts offer another key benefit. When a system notices suspicious activity, alerts surface immediately, enabling a rapid investigation. If further clarity is needed, Glassbox’s unique capabilities help teams replay user sessions and trace suspicious signals back to their source across digital environments.
Real-World Use Cases of Machine Learning in Fraud Detection
Organizations that prioritize AI-driven fraud detection gain a significant advantage by spotting suspicious behavior in a broad range of digital interactions. From conventional credit card scams to advanced account takeovers, machine learning systems offer the flexibility and speed needed to keep fraud at bay.
Here are some of the most common real-world scenarios where these solutions excel:
Credit Card Fraud Detection: By correlating transactions across multiple geographies and channels, ML algorithms uncover patterns that deviate from a cardholder’s established spending habits. This drives immediate alerts before criminals can finalize large unauthorized purchases.
Bot and Automation Fraud: Automated scripts can rapidly abuse promo codes or perform credential stuffing attacks. ML-enabled systems pick up on strange traffic spikes or repeat purchase patterns, distinguishing bots from genuine users. This prevents coupon abuse and account breaches orchestrated by fraudsters operating at scale.
Synthetic Identity Fraud: Criminals sometimes blend stolen and fabricated details to create entirely new identities. Machine learning models detect inconsistencies in behavior and usage that raise red flags, even when the identity looks legitimate on the surface.
Account Takeover Prevention: Whether it’s through brute force attempts or stolen credentials, fraudsters aim to seize control of valuable accounts. ML processes signals like repeated login failures or strange session behaviors, blocking unauthorized access before it spreads.
Insider Threats and Loan or Insurance Claim Manipulation: Fraud can come from employees or partners with established access. Machine learning tracks user interactions and notes anomalies, helping you detect potential policy changes or suspicious transaction approvals from internal sources.
Real-Time Tampering: Hackers often modify form fields, input data or underlying DOM elements to alter transaction amounts. AI systems flag these manipulations by comparing the intended user flow against actual traffic. Glassbox’s visibility into every session highlights abnormal field edits in the moment.
Suspicious Field-Level Editing and Coupon Abuse: Some fraud rings operate by repeatedly testing discount codes or layering rebates to get bigger payouts. Machine learning quickly spots repeated coupon use and the attempts to exploit promotional loopholes.
Proactive Fraud Detection With Glassbox
Machine learning enables financial institutions to catch fraud in real time—even at massive transaction scales. Manual reviews can’t keep pace, but AI models pinpoint fraud indicators instantly, letting teams act before losses mount. In a landscape where fraud tactics shift hourly, speed is survival.
Glassbox delivers unmatched visibility into sophisticated schemes. By reconstructing digital journeys in real time, it reveals exactly how fraud unfolds—tracking every user action until the moment of attack. This granular data exposes where anomalies start and how to neutralize them fast.
While most tools rely on account, device or transaction IDs, Glassbox digs deeper. It ties granular session data—steps, context, behaviors—to each journey ID. This exposes cross-account bot attacks or multi-device fraud rings that ID-based systems miss. AI algorithms then flag behavioral red flags, prompting immediate investigation.
Key Glassbox features:
Anomaly Detection: Flags rapid form fills, hidden field tampering and other suspicious patterns and bot-like behavior
Cross-Channel Insights: Connects fraud signals across web, mobile and contact center touchpoints
Compliance-First Design: Auto-masks sensitive data (PII, passwords) during analysis to meet regulations
Unlike basic monitoring tools, Glassbox’s GIA isolates fraud at scale by analyzing both suspicious and legitimate journeys. The result? Fewer false alarms, faster triage and unwavering trust in your decisions.
As fraud evolves, Glassbox adapts—continuously refining detection models without disrupting customer experiences. Teams stay ahead with insights that update as fast as the threats do.
Harness the Power of AI for Fraud Prevention
Machine learning has rapidly become the cornerstone of modern fraud prevention, delivering real-time detection and finely tuned protection. By analyzing digital interactions from multiple angles, algorithms uncover patterns of suspicious activity that might otherwise be missed. This adaptive, AI-driven approach keeps financial services providers a step ahead of evolving threats, reinforcing trust and safeguarding revenue.
If you’re looking to strengthen your defenses, explore how Glassbox’s advanced analytics can enhance your current fraud detection and compliance initiatives. Take a tour of the platform today.